Machine Learning Reveals New Insights
Atypical ductal hyperplasia, a particular kind of breast lesion, is associated with a four- to five-fold increase in the risk of breast cancer. Currently, surgical removal is recommended whenever these lesions are detected—but only 20% to 30% of these cases progress to cancer.
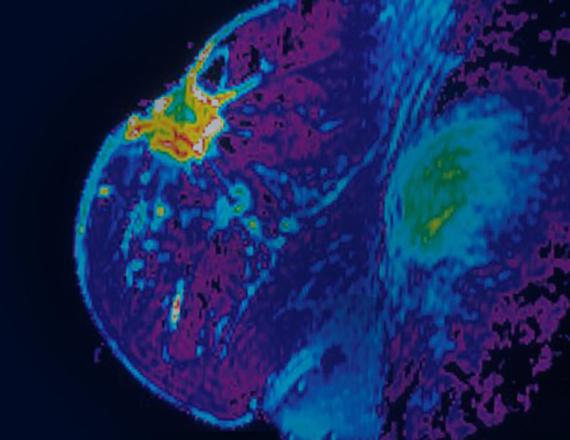
Saeed Hassanpour, PhD, is finding innovative ways to apply machine learning—the use of algorithms and statistical models by computers to detect patterns and make inferences—to a variety of fields, including breast cancer treatment and behavioral health care. Hassanpour and his research team developed a machine learning method that can identify with 98% accuracy which cases of atypical ductal hyperplasia are malignant prior to removal, thus sparing from surgery some women who would otherwise have undergone a costly and invasive operation for what would ultimately prove to be a benign lesion.
Hassanpour is an associate professor of biomedical data science and epidemiology at the Geisel School of Medicine and a member of the Cancer Population Sciences Research Program at Norris Cotton Cancer Center. Photo by Rob Strong.
In the field of behavioral health, Hassanpour and a team of researchers are developing a promising new approach for identifying substance use risk through the combined use of machine learning and social media data. In their study, nearly 2,300 adult participants completed a survey about their substance use while allowing researchers to extract numerous images, captions, and comments from their Instagram posts. The model proved able to detect risk for alcohol use with high accuracy. Further efforts by Hassanpour’s team will target tobacco, prescription drug, and illicit drug use, and also incorporate social network platforms including Facebook and Twitter.
Other work in Hassanpour’s lab focuses on using radiology images to aid in lung, esophageal, and colorectal cancer classification and in detection of osteoporotic fractures. Additional behavioral health research aims to predict depression risk in social media users based on community responses to their posts.